Understanding the Fundamentals of Business Data Collection
The Importance of Data Collection
Did you know that 94% of businesses say data is essential to their growth strategy, yet only 44% are fully equipped to collect it effectively? In today’s digital landscape, the ability to gather and analyze business data isn’t just an advantage – it’s a necessity
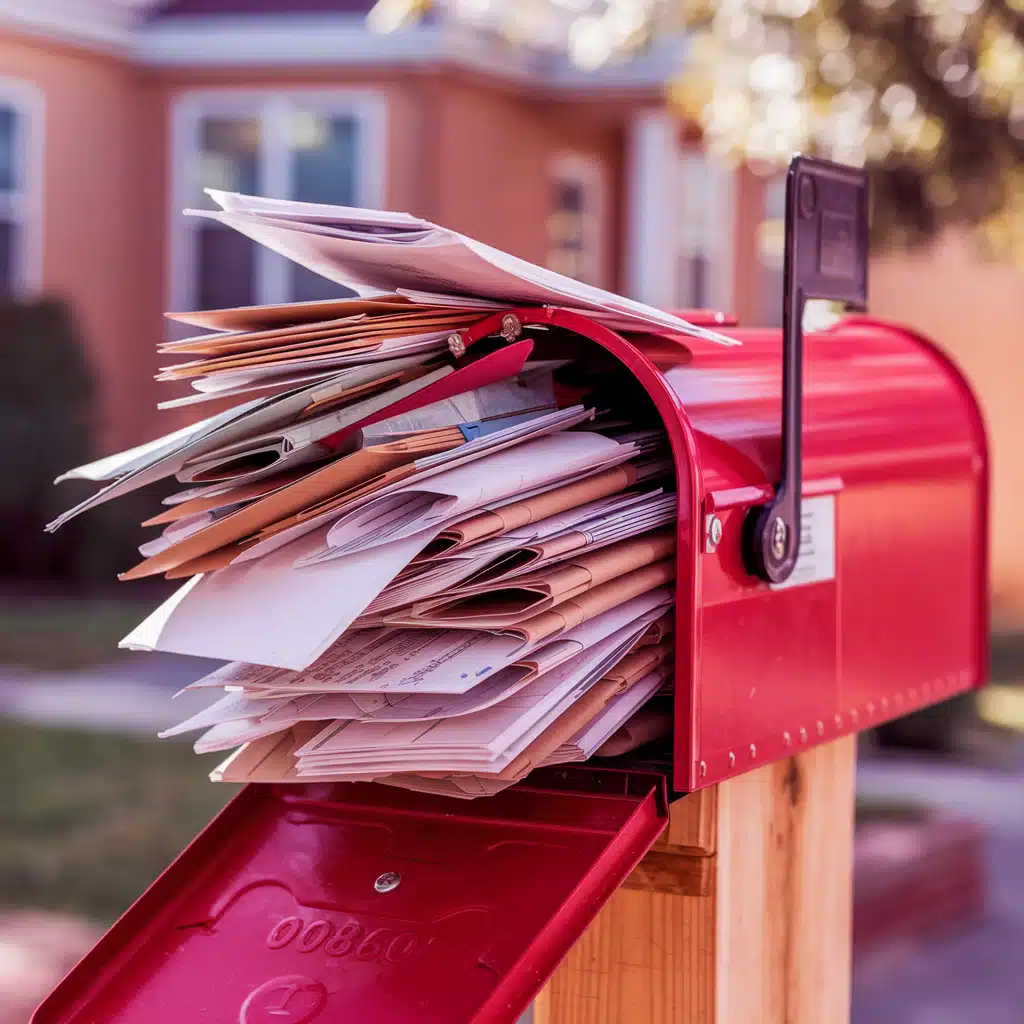
I’ve come to appreciate the power of well-executed traditional methods. While they might not sound as exciting as cutting-edge approaches for customer data collection, these foundational techniques have consistently delivered reliable insights that drive business success.
For more details on conducting business research, check out my comprehensive guide “How to Do Business Research: A Step-by-Step Guide (2025)“
Traditional Data Collection Methods
- Customer Survey Distribution: The art of crafting perfectly timed surveys that capture genuine feedback without survey fatigue. I’ve seen response rates triple simply by optimizing delivery timing and question flow.
- Sales Data Analysis: Systematic collection of transaction data that reveals purchasing patterns. One of our biggest breakthroughs came from analyzing transaction timestamps alongside product categories.
- Website Analytics Implementation: Going beyond basic Google Analytics to track micro-conversions and user journeys. We uncovered a goldmine of insights by tracking scroll depth and exit intent patterns.
- Social Media Engagement Tracking: Real-time data collection monitoring that goes beyond likes and shares, diving deep into conversation threads and sentiment patterns. The real insights often hide in the comment timing and interaction sequences we capture as they happen.
- Email Response Analysis: Sophisticated data validation techniques applied to email campaign performance, including time-to-open patterns and device preference analysis.
- Point-of-Sale System Integration: Detailed user behavior tracking through in-store purchase data, including basket analysis and inventory movement patterns. By monitoring customer interactions in real-time, this helps us to predict inventory needs with remarkable accuracy.
- Customer Service Log Analysis: Systematic categorization and analysis of support tickets and customer interactions. The patterns we found in support request timing transformed our staffing approach.
- CRM Data Collection: Structured gathering of customer lifecycle data, from first touch to ongoing engagement. The relationship patterns we uncovered changed our entire approach to customer segmentation.
- Form Submission Tracking: Detailed analysis of how users interact with online forms, including abandonment patterns and completion rates. Small tweaks based on this data led to massive conversion improvements.
- Loyalty Program Data Collection: Using big data collection methods to systematically track customer rewards program participation and redemption patterns.
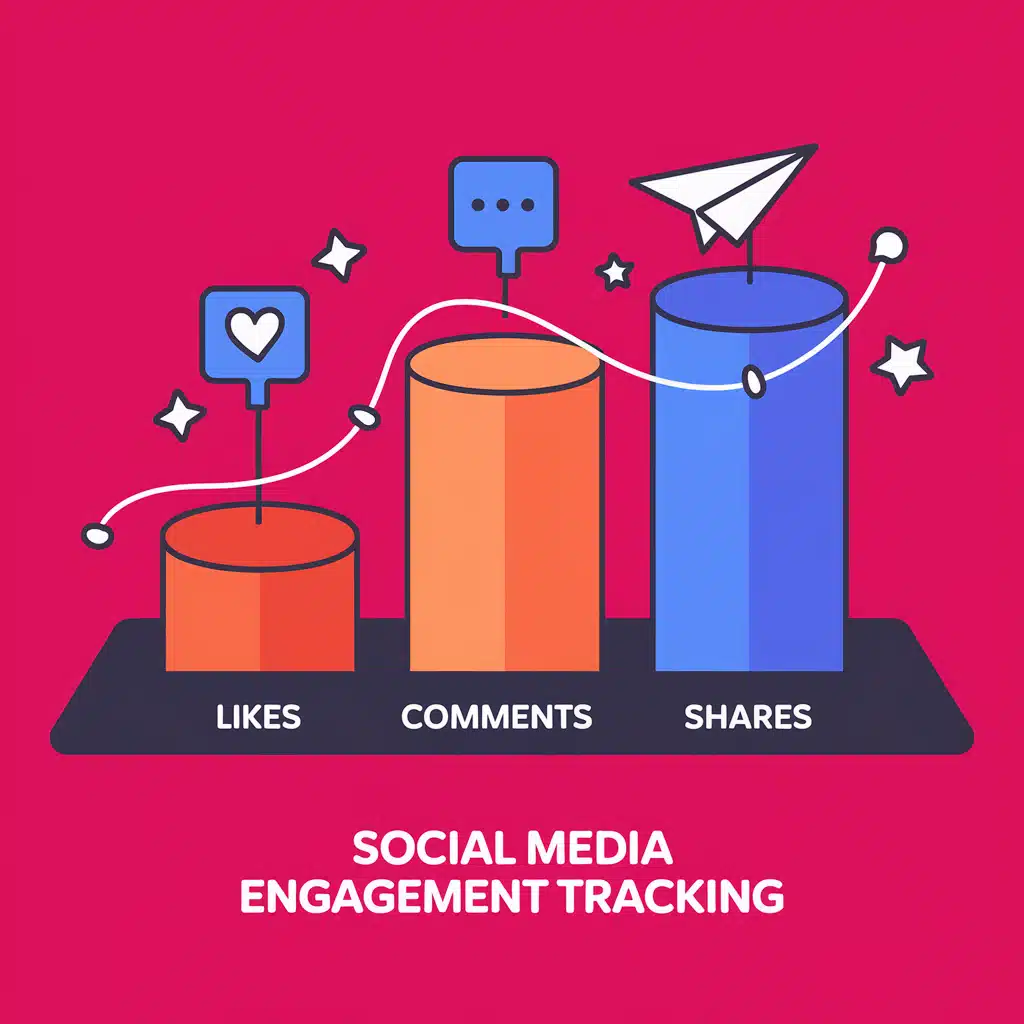
These methods might seem ordinary, but when implemented with precision and attention to detail, they create the robust foundation necessary for more advanced analytics. They’re like the reliable instruments in an orchestra – essential for creating the perfect symphony of data insights.
But wait until you hear about the cutting-edge methods that take these foundations to the next level…
Building on those foundational business data collection methods, I’ve discovered some mind-blowing data collection techniques that most analysts haven’t even heard of yet. These innovative approaches have transformed how we understand business intelligence, taking those traditional methods into the future.
Cutting-Edge Methods in Data Collection
Physical Space Analysis
- Neuroarchitectural Pattern Mapping: We started combining store layout data with advanced AI-powered spatial sensors. This revealed how subtle changes in building design unconsciously influence both employee collaboration and customer purchase decisions.
- Ambient Intelligence Networks: Think of it as POS system integration on steroids. We’ve deployed microscopic sensors throughout retail spaces that create 3D behavior maps based on the tiniest environmental changes caused by human presence.
- Social Gravity Analysis: Moving beyond basic social media tracking, we’re measuring the invisible pull between customers in physical spaces. The group decision dynamics we’ve uncovered have revolutionized our understanding of social influence.
Advanced Behavioral Analysis
- Biometric Resonance Tracking: Remember those customer service logs? Well, we’ve elevated that by using thermal imaging and electromagnetic field analysis to measure real emotional engagement during product interactions.
- Chronometric Experience Sampling: We’ve evolved from basic form submission tracking to capturing micro-moments of customer interaction using AI-powered temporal analysis.
- Psychological Weather Mapping: This takes email response analysis into new territory by correlating atmospheric conditions with consumer behavior.
Quantum Computing Applications
- Quantum Time-Series Analysis: Imagine taking those loyalty program patterns I mentioned and analyzing them across multiple parallel universes of data streams.
- Quantum Behavioral Fingerprinting: Think of this as website analytics meeting quantum computing. We’re analyzing the unique ways individuals interact with digital interfaces at a quantum level.
Digital Intelligence
- Neural Transaction Analysis: Taking traditional sales data analysis to the next level, we’re using machine learning to decode subtle psychological patterns in payment timing and frequency.
- Digital Echo Mapping: We’ve moved beyond basic website analytics to analyze the unique “digital exhaust” signatures people leave across devices.
The Power of Integration
What fascinates me most is how these advanced methods don’t replace traditional techniques – they enhance them. Every traditional method I mentioned earlier becomes exponentially more powerful when combined with these cutting-edge approaches. We’re not just collecting data anymore; we’re uncovering the hidden patterns that shape business outcomes.
Integration Examples
The real magic happens in the integration. For example, combining traditional CRM data with Ambient Intelligence Networks gives us a complete picture of customer behavior that neither method could provide alone. It’s like having x-ray vision into customer decision-making processes.
Future Implications for Business Data Collection
These methods might sound like science fiction, but they’re already transforming how forward-thinking businesses gather intelligence. The future of data collection isn’t just about gathering more data – it’s about gathering smarter data in ways we never imagined possible.
Data Collection Methodologies
The Process
Here’s what I’ve learned about primary data collection – it’s like cooking from scratch. You get exactly what you want, but it takes more time and resources. I’ve used everything from online surveys to focus groups, and each method has its sweet spot. Customer interviews gave us the richest insights, but they were super time-consuming. Online surveys were easier to scale, but getting meaningful responses? That’s another story.
*Pro Tip*: offering a small incentive boosted our response rates by nearly 40%.
Secondary data collection, on the other hand, is like getting a pre-made meal. It’s faster and often cheaper, but you might not get exactly what you’re looking for. I’ve spent countless hours digging through government databases, industry reports, and competitor analyses. The trick is knowing where to look – the U.S. Census Bureau and Bureau of Labor Statistics have been goldmines for demographic and economic data.
When it comes to choosing between quantitative and qualitative approaches, I learned (the hard way) that it’s not an either-or situation. Quantitative data tells you the “what” – like our customer satisfaction scores dropping 15% in Q2. But qualitative data tells you the “why” – those detailed customer comments about our new interface being confusing were pure gold for our product team.
Listen, we need to talk about the elephant in the room – data privacy laws. GDPR and CCPA aren’t just fancy acronyms to throw around at meetings. Trust me, you don’t want to learn about compliance the expensive way. Some key things to remember:
Data Privacy and Compliance
Key Privacy Requirements
- Always get explicit consent before collecting personal data
- Be crystal clear about how you’re going to use the information
- Give people an easy way to access or delete their data
- Document everything about your data collection processes
One thing that totally changed my approach was implementing data collection frameworks. Start with your business objectives and work backward. I created a simple matrix that matches business goals with appropriate data collection methods. For example, if you need to understand customer satisfaction, a mix of NPS surveys (quantitative) and follow-up interviews (qualitative) works wonders.
Best Practices and Lessons Learned
The most important lesson? Data collection isn’t just about gathering information – it’s about gathering the RIGHT information. I wasted three months collecting data that looked great on paper but didn’t actually help us make any decisions. Now I always ask, “How will this data help us solve a specific business problem?”
And here’s something that might surprise you – sometimes less data is more. I’ve seen teams get paralyzed by analysis because they collected too much irrelevant data. Focus on quality over quantity, and make sure every piece of data you collect ties back to your business objectives.
Just remember to stay flexible with your methods. What works for one business question might not work for another. And always, always keep an eye on those privacy regulations – they’re constantly evolving, and staying compliant is crucial for maintaining customer trust.
Traditional Business Data Collection Methods
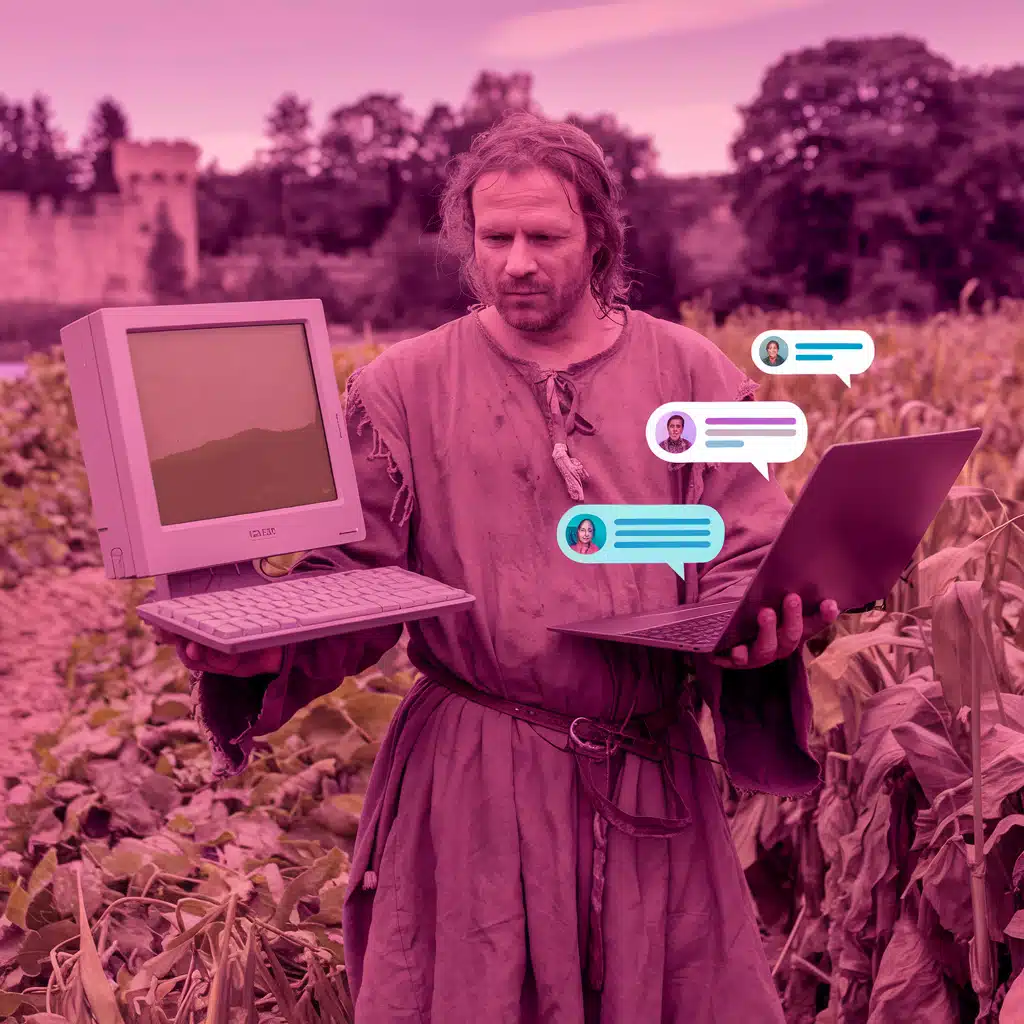
Let me share what I’ve discovered about traditional data collection methods. It’s funny – when I first started out, I thought creating a survey was as simple as writing down a few questions and hitting send. That misconception led to some pretty useless data!
Survey Design and Implementation
Speaking of surveys, let’s talk about what actually works. I spent months refining customer feedback surveys, and the biggest game-changer was question sequencing. Starting with easy, non-threatening questions before diving into the deeper stuff made a huge difference in completion rates. You wouldn’t believe how much our response rate jumped – from a measly 12% to over 35% – just by restructuring the flow.
Here’s something that drove me nuts at first: survey bias. I kept getting overly positive responses that didn’t match our customer service tickets at all. Turns out, our questions were totally leading the witnesses! Instead of asking “How great was your experience?” (yeah, I really did that), I learned to use neutral language like “How would you describe your experience?” The difference in the quality of feedback was like night and day.
Focus Groups and Interviews
When it comes to interviews and focus groups, I learned some tough lessons. My first focus group was a disaster – I had a chatty participant who dominated the whole conversation while others barely spoke. Now I always use a structured approach with clear time limits for each response, and I’m not shy about politely redirecting the conversation when needed. Pro tip: sending discussion topics to participants beforehand helps them prepare more thoughtful responses.
Documentation Analysis
Documentation analysis – now that’s something that took me a while to appreciate. I used to think it was just about reading through old reports, but it’s so much more strategic than that. I developed a system where we track patterns in customer complaints, service requests, and internal reports.
Observational Research
For observational data, you’ve got to be really systematic about it. Now I use a structured observation checklist focused on specific behaviors or processes. For example, when studying customer flow in stores, we tracked things like:
Key Observation Points
- Time spent in different departments
- Common walking patterns
- Points where customers seemed confused
- Interactions with staff members
Method Integration and Documentation
One of my biggest revelations was about combining these methods effectively. Each approach has blind spots. Surveys might tell you what people say they do, but observation shows what they actually do. And sometimes, the gold is in the gaps between these different data sources.
Documentation has saved my bacon more times than I can count. Early on, I was pretty casual about recording my data collection processes. Big mistake! When we needed to verify some findings months later, we had to redo entire sections of research because we couldn’t prove our methods were consistent. Now I document everything – sampling methods, question revisions, even the time of day we conduct observations.
Tools and Technology
Let’s talk about tools because they matter more than you might think. I wasted a lot of time using basic survey tools that couldn’t handle our analysis needs. Investment in proper research software paid for itself within months through time saved and better insights. For focus groups, good recording equipment is worth its weight in gold – trying to rely on handwritten notes alone is a recipe for missed insights.
Remember, these traditional methods might seem old-school compared to fancy digital analytics, but they’re incredibly powerful when done right. The key is being systematic, thorough, and always keeping your business objectives in sight.
Digital and Automated Data Collection Techniques
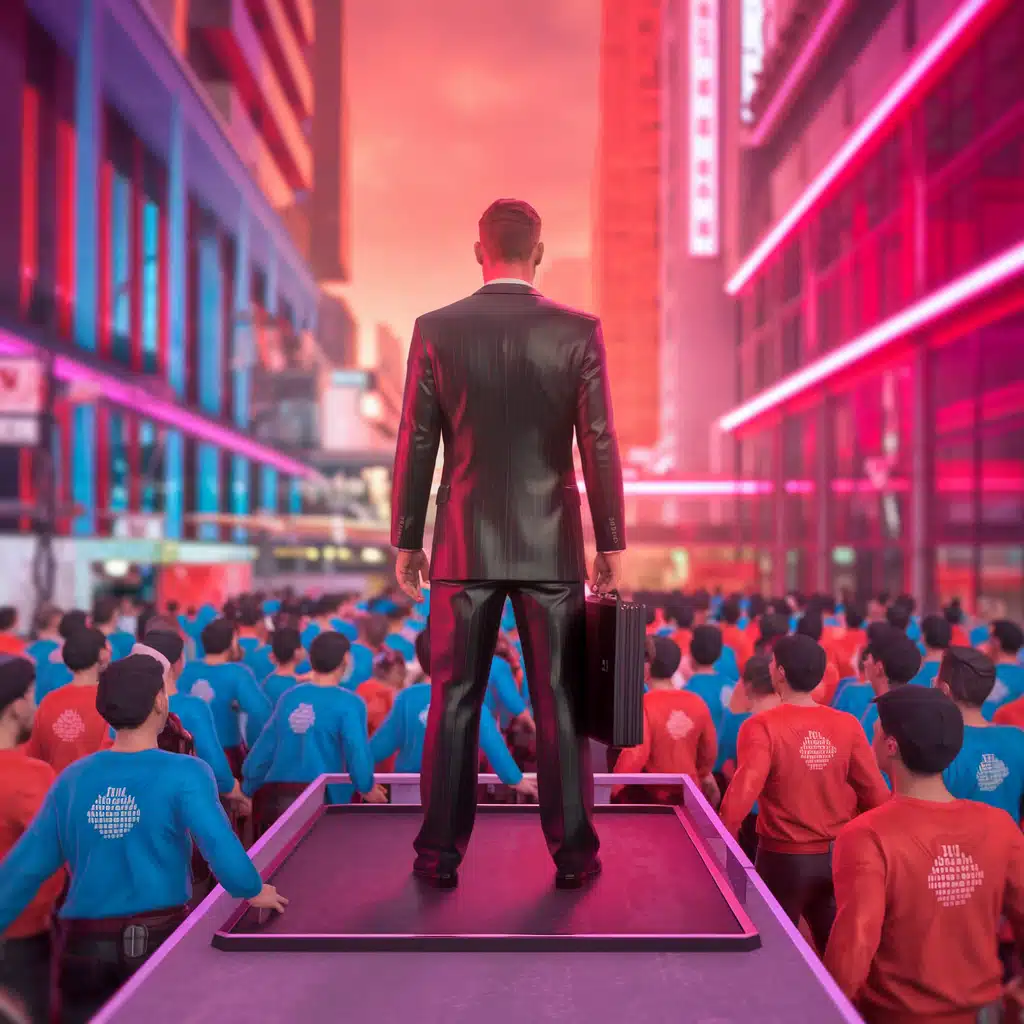
Digital Data Collection Journey
When I first dove into web analytics, I felt like a kid in a candy store with way too many options. Google Analytics was throwing numbers at me left and right, and I had no clue what really mattered.
Web Analytics Implementation
Strategic Focus
The turning point came when I realized I was tracking everything but understanding nothing. Here’s what actually worked: starting with specific business questions. Instead of drowning in data, we identified key performance indicators that actually meant something. Bounce rates, conversion funnels, user paths – these became our bread and butter for making real decisions.
Tracking Code Lessons
We thought we had everything set up perfectly until we discovered six months of mobile traffic wasn’t being tracked properly because of a tiny code snippet error. Now I swear by regular data verification – checking our tracking at least monthly across different devices and browsers. Trust me, it saves so many headaches down the road.
Social Media Intelligence
Social media monitoring was another eye-opener. At first, we were just counting likes and shares (rookie mistake!). Then we started using sentiment analysis tools, and wow – the insights were game-changing. But here’s the thing about automated sentiment analysis: it’s not perfect with sarcasm or cultural nuances. We learned to use a hybrid approach, combining automated tools with human review for our most important social conversations.
CRM Systems and Automation
Speaking of automation, let me tell you about our CRM journey. The biggest mistake I see companies make is treating their CRM like a fancy address book. We transformed ours into a powerhouse of customer insights by:
- Setting up automatic tracking of all customer touchpoints
- Creating custom fields for industry-specific data points
- Implementing automated tagging based on customer behaviors
- Setting up regular data cleaning protocols
Our data quality improved dramatically once we established strict input standards. Garbage in, garbage out isn’t just a saying – it’s the hard truth of digital data collection.
The integration piece is super important. I learned that having different systems that don’t talk to each other is almost worse than having no systems at all. We spent months building APIs and data pipelines to connect our web analytics, CRM, and social media monitoring tools. It was a pain, but having a unified view of our customer journey made it worth it.
Privacy and Security
Privacy and security in digital collection is no joke. After a small data breach scare (thankfully caught early), we implemented much stricter protocols. Every automated system now has built-in privacy checks, and we regularly audit our data collection processes. With regulations like GDPR and CCPA, you can’t be too careful.
One really cool discovery was using heat mapping tools on our website. Seeing exactly where users clicked, stopped scrolling, or got stuck completely changed our web design approach. We found that some of our most important content was sitting in what we now call the “dead zones” – areas users rarely scrolled to.
The biggest game-changer? Real-time alerting systems. We set up automated triggers for unusual patterns in our data – sudden traffic spikes, dramatic changes in social sentiment, or drops in conversion rates. It’s like having a 24/7 monitoring team without the overhead.
And here’s something not enough people talk about: data storage costs. When we first started with IoT sensors, we were collecting data every second because we could. Turned out we only needed 5-minute intervals for meaningful analysis, and the change saved us a fortune in storage costs.
Advanced Technology in Business Data Collection
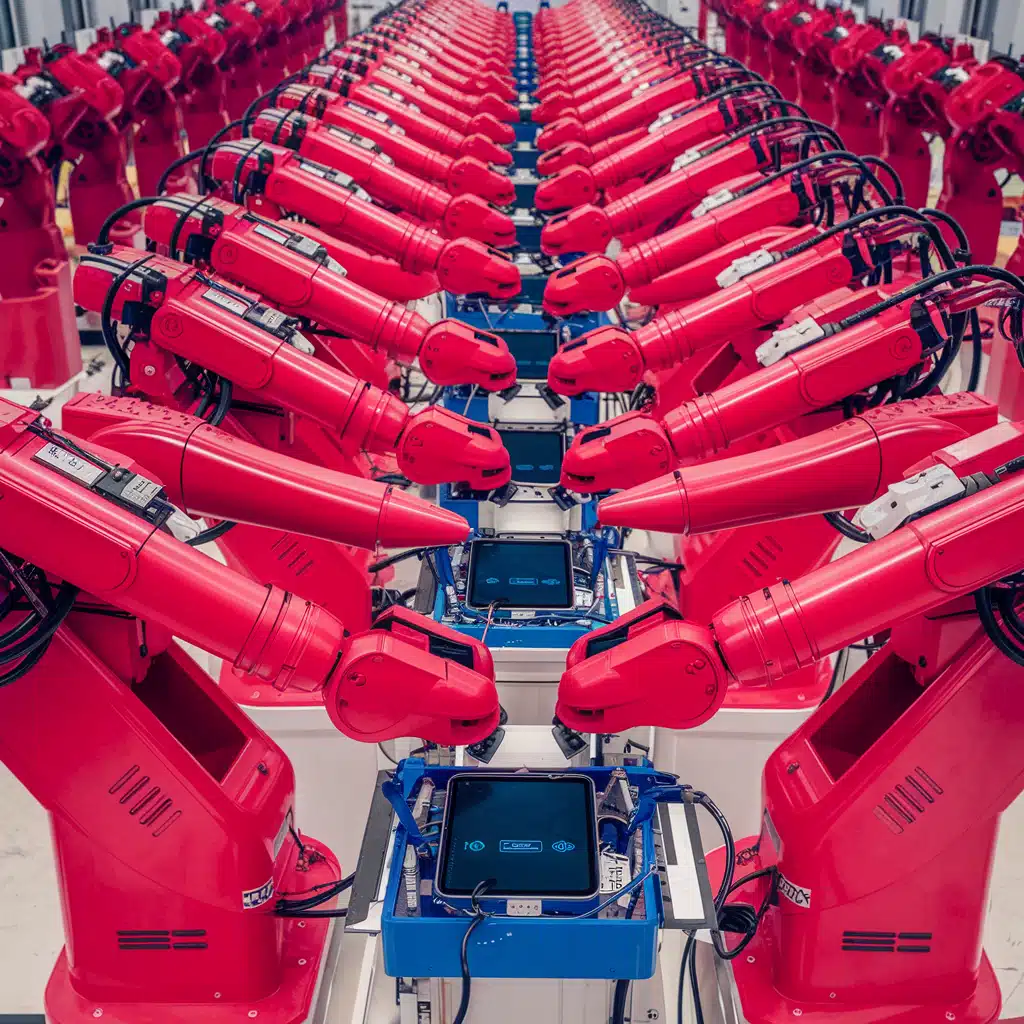
My journey into advanced data collection technology started with a rather humbling experience. I thought implementing AI for data gathering would instantly solve all our problems – spoiler alert: it definitely didn’t! But what I learned along the way completely transformed how I approach modern data collection.
Machine Learning Implementation
The first time I worked with machine learning for data collection, I made the classic mistake of throwing technology at a problem before fully understanding it. Our AI system was collecting massive amounts of customer interaction data, but we hadn’t properly defined what patterns we were looking for. After that expensive lesson, I learned to start with clear objectives before implementing any advanced tech.
AI Implementation Strategy
Speaking of automation, here’s a crucial tip about AI implementation: start small. Our first AI data collection project focused just on categorizing customer service emails. Once we got that working smoothly, we expanded to more complex applications. The key was building confidence and expertise gradually rather than trying to boil the ocean.
Mobile data collection taught me some hard lessons about user experience. We built this fancy data collection app with all the bells and whistles, but our field teams hated it because it drained their phone batteries too quickly. Sometimes simpler really is better – we rebuilt it with basic functions and got much better adoption.
Data Stream Management
Managing big data streams requires serious strategy. I developed a three-tier approach:
- Hot data: Frequently accessed, needs immediate availability
- Warm data: Occasionally accessed, can have slight retrieval delay
- Cold data: Rarely accessed, can be heavily compressed and archived
Real-time processing came with its own challenges. We had to completely rethink our data validation processes. Traditional batch processing let us clean data before analysis, but with real-time streams, we needed automated validation rules running constantly. Getting those rules right took months of refinement.
The intersection of AI and mobile data collection has been particularly interesting. We implemented machine learning algorithms that adapt to individual user behavior patterns, making data collection more efficient over time. For example, our system learned to predict which data fields different users typically need, streamlining their input process.
System Integration
The most valuable lesson? Integration is everything. Having advanced technology for data collection is great, but if it doesn’t play nice with your existing systems, you’re just creating expensive data silos. We now spend almost as much time planning integration strategies as we do implementing new collection methods.
Conclusion
The landscape of business data collection is constantly evolving, with new technologies and methods emerging regularly. By implementing a mix of traditional and modern data collection methods, you can create a robust data strategy that drives your business forward. Remember, the key isn’t just collecting data – it’s collecting the right data in the right way. Start with one or two methods that align with your business goals, and gradually expand your data collection toolkit as your needs grow.
What is data collection, and why is it important for businesses?
Data collection is the systematic gathering of information to inform business decisions. It’s crucial because 94% of businesses say data is essential to their growth strategy, helping them understand customer behavior, improve operations, and make informed decisions.
What’s the difference between primary and secondary data collection?
Primary data is collected firsthand (like surveys and interviews), giving you exactly what you need but requiring more resources. Secondary data comes from existing sources (like government databases or industry reports), being faster to obtain but potentially less specific to your needs
Which traditional data collection methods are most effective?
The most effective methods typically include:
Customer surveys (when properly designed)
Sales data analysis
Website analytics
Customer service logs
Point-of-sale system integration
How can I improve survey response rates?
You can improve survey response rates by:
Starting with easy, non-threatening questions
Using neutral language to avoid bias
Offering small incentives (can boost rates by up to 40%)
Optimizing survey timing and length
What are some cutting-edge data collection methods?
Modern approaches include:
Neuroarchitectural Pattern Mapping
Ambient Intelligence Networks
Biometric Resonance Tracking
Digital Echo Mapping
Quantum Behavioral Fingerprinting
What are the key privacy considerations in data collection?
Essential privacy considerations include:
Getting explicit consent before collecting personal data
Clearly communicating how data will be used
Providing data access and deletion options
Maintaining proper documentation
Complying with regulations like GDPR and CCPA
How often should I review and update my data collection methods?
Regular reviews should occur:
Monthly for tracking code verification
Quarterly for method effectiveness
Annually for overall strategy
Whenever regulations change
When new technologies emerge